Tracking Bias in Automated Decision Analytics: Understanding and Addressing Challenges
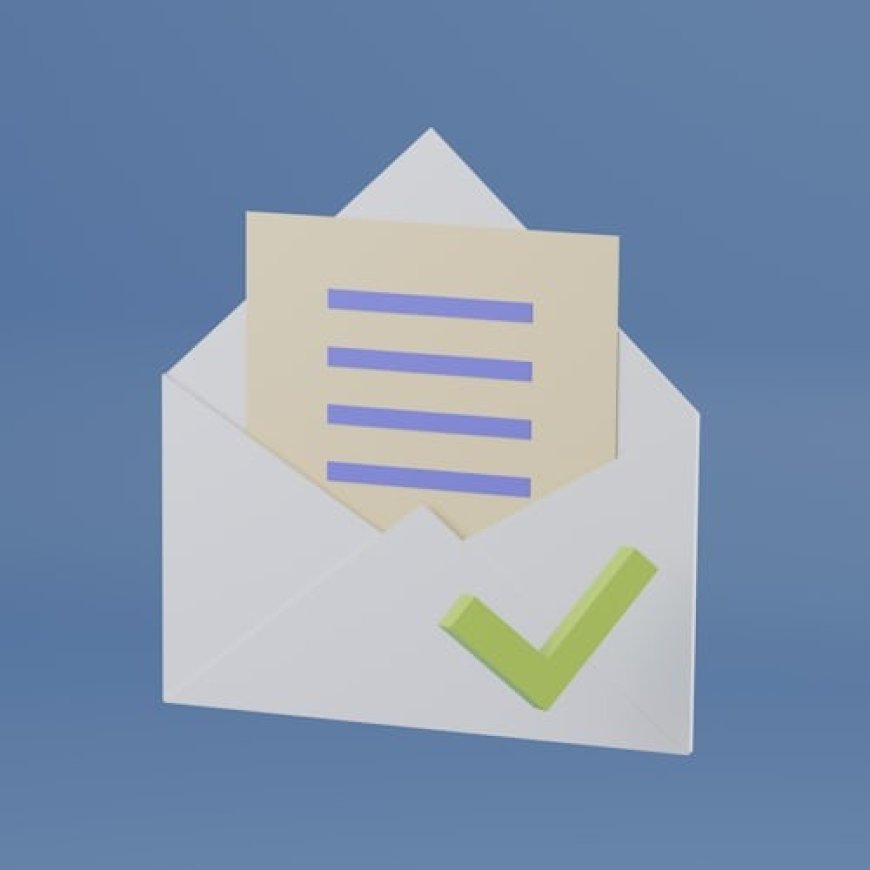
In recent years, AI-powered analytics have become integral in decision-making processes across various industries. From finance to healthcare, businesses leverage artificial intelligence (AI) to analyze data, predict trends, and make critical decisions. However, as these AI systems evolve, they often inherit biases present in the data they are trained on. Tracking bias in automated decision analytics is essential to ensure fairness, accuracy, and transparency in AI-driven decisions. This article explores the impact of bias on AI analytics, why it matters, and how organizations can detect and mitigate these biases for better outcomes.
The Role of AI-Powered Analytics in Decision-Making
AI-powered analytics refers to the use of artificial intelligence algorithms to analyze and interpret data for decision-making. These systems are designed to process large datasets at scale, uncover patterns, and provide actionable insights faster and more accurately than human analysts. For example, AI can help predict customer behavior, optimize supply chain logistics, or evaluate risk in financial transactions.
While the potential benefits are vast, the reliance on automated decision systems introduces risks—chief among them, the possibility of bias. AI systems learn from historical data, which may reflect biases inherent in society, such as racial, gender, or socioeconomic biases. If not carefully monitored, these biases can lead to unfair or discriminatory decisions, which can have severe consequences.
What is Bias in Automated Decision Analytics?
Bias in AI-powered analytics occurs when algorithms produce outcomes that are systematically unfair or skewed due to prejudices in the training data. In the context of automated decision analytics, bias can manifest in various ways:
-
Data Bias: If the data used to train an AI model is incomplete or unrepresentative, the algorithm will learn from these imperfect inputs. For example, an AI model trained on biased data may inaccurately predict loan eligibility for individuals from certain racial or ethnic groups.
-
Algorithmic Bias: Even with unbiased data, the way an AI algorithm processes and interprets that data can introduce bias. This can happen if the algorithm prioritizes certain features over others, leading to skewed outcomes.
-
Human Bias: The people designing AI systems may unintentionally embed their own biases into the decision-making models. This type of bias can be difficult to detect, as it originates from human assumptions, beliefs, or preferences.
Why Tracking Bias in Automated Decision Analytics is Critical
Understanding and addressing bias in automated decision-making systems is crucial for several reasons. First and foremost, biased AI decisions can lead to discrimination and unfair treatment of individuals or groups. For instance, a biased hiring algorithm might favor candidates of a particular gender or ethnicity, leading to inequality in job opportunities. Similarly, biased credit scoring models could unfairly deny loans to certain demographics.
In addition to the ethical concerns, biased AI decisions can damage an organization’s reputation. Businesses that rely on AI-powered analytics for customer-facing operations risk alienating customers if their systems produce unfair outcomes. Regulatory scrutiny is also increasing around the use of AI, and companies that fail to address bias may face legal consequences.
Tracking bias allows organizations to identify where these issues arise and take corrective measures. It also helps improve the overall accuracy and reliability of AI systems, ensuring that decisions are based on fair and equitable standards.
The Types of Bias That Can Affect AI-Powered Analytics
Various types of bias can affect the accuracy and fairness of AI-powered analytics. Some of the most common include:
1. Sampling Bias
Sampling bias occurs when the data used to train an AI model is not representative of the entire population. For example, if a facial recognition system is trained primarily on images of light-skinned individuals, it may struggle to accurately identify people with darker skin tones. This can lead to erroneous or discriminatory outcomes, especially when the system is deployed in real-world applications.
2. Label Bias
Label bias happens when human annotators label data in a way that reflects their own biases. For example, if a dataset for training a job applicant screening algorithm contains labels that reflect societal biases, the AI system will likely inherit these biases. This can perpetuate harmful stereotypes and create unfair systems that disproportionately disadvantage certain groups.
3. Measurement Bias
Measurement bias arises when the tools used to collect data are inaccurate or imprecise. For instance, if a healthcare AI system relies on medical records that are incomplete or contain errors, the resulting analysis may be biased, leading to incorrect diagnoses or treatment recommendations.
4. Exclusion Bias
Exclusion bias occurs when certain groups are not adequately represented in the training data. This can happen when certain demographics, such as elderly individuals or people with disabilities, are excluded from datasets. The AI system may then fail to accurately predict outcomes for these underrepresented groups.
Techniques for Tracking and Identifying Bias in AI Models
To ensure that AI systems remain fair and unbiased, businesses and organizations must implement strategies to track and identify bias throughout the lifecycle of an AI-powered analytics model. Below are some effective techniques:
1. Audit and Transparency
Regularly auditing AI models for bias is a crucial step in tracking bias. This involves reviewing the training data, examining how algorithms process that data, and testing the outcomes for fairness. Transparency in the decision-making process is essential to understanding how AI models arrive at their conclusions. Open algorithms and clear documentation can help identify biases and improve accountability.
2. Bias Detection Tools
Several bias detection tools and frameworks are available to help organizations analyze and mitigate bias in AI systems. Tools like Fairness Indicators, AI Fairness 360, and What-If Tool allow developers and data scientists to assess fairness metrics, test different demographic groups, and evaluate model performance across various datasets.
3. Diverse Data Collection
To avoid data biases, organizations should ensure that the data they use to train AI systems is diverse and representative of all relevant populations. This may involve collecting additional data from underrepresented groups or rebalancing datasets to ensure that different perspectives are included in the model's training process.
4. Cross-Disciplinary Collaboration
Collaboration between data scientists, ethicists, legal professionals, and sociologists is key to identifying and mitigating bias. A multidisciplinary team can help ensure that AI models consider fairness from various angles, including legal compliance, social equity, and ethical implications.
5. Bias Mitigation Algorithms
Once biases are detected, businesses can implement bias mitigation techniques to reduce their impact. These can include adjusting the training data, modifying the model's structure, or using algorithms specifically designed to reduce bias, such as fairness constraints during training.
The Role of Regulation and Ethical Standards in Bias Detection
As AI-powered analytics become more widespread, governments and regulatory bodies are stepping in to ensure that AI systems are fair and non-discriminatory. In some regions, such as the European Union, there are regulations that require businesses to assess and mitigate bias in AI systems. For instance, the EU’s Artificial Intelligence Act aims to regulate high-risk AI systems, including those used in decision-making processes.
Additionally, organizations are encouraged to adopt ethical frameworks for AI development. The principles of fairness, accountability, transparency, and ethics (FATE) are commonly used in AI development to guide practitioners in creating responsible systems. Adhering to these guidelines can help reduce bias and improve the trustworthiness of AI-powered analytics.
Steps to Mitigate Bias in Automated Decision Analytics
While bias may never be entirely eliminated, businesses can take steps to reduce its impact on AI-powered analytics. These steps include:
1. Ensuring Diversity in Training Data
To prevent bias from creeping into AI systems, it’s crucial to ensure that training data is diverse and inclusive. This involves proactively collecting data from different demographic groups and ensuring that the data reflects the full spectrum of potential real-world scenarios.
2. Implementing Fairness Metrics
Organizations should incorporate fairness metrics into their AI models to track the fairness of decisions made by the system. These metrics can be used to evaluate whether certain groups are being unfairly impacted by automated decisions.
3. Continuous Monitoring
Tracking bias is not a one-time activity. AI models should be continuously monitored after deployment to ensure they remain unbiased as new data is introduced. This ongoing monitoring allows businesses to identify any emerging biases and make necessary adjustments.
4. Providing Human Oversight
Although AI can provide valuable insights, human oversight remains essential to ensure that decisions are fair and ethical. Combining AI analytics with human judgment helps prevent the unchecked application of biased systems.
Moving Towards Fair and Transparent AI-Powered Analytics
Addressing bias in automated decision analytics is not only crucial for ensuring fairness and equity, but it also strengthens the credibility and effectiveness of AI systems. By tracking and mitigating bias, businesses can make better, more accurate decisions, while fostering trust with customers and stakeholders.
As AI continues to evolve, organizations must remain vigilant about the potential risks associated with bias. Through thoughtful design, rigorous testing, and ethical practices, we can ensure that AI-powered analytics serve everyone equitably, driving progress and innovation in a fair and just manner.
Final Thoughts on Tackling Bias in AI Systems
The effort to track and reduce bias in automated decision analytics is ongoing, but the benefits are clear. Fairer, more accurate AI systems help prevent discrimination, enhance decision-making, and build trust with users. As AI becomes a more integral part of our daily lives, ensuring that it operates without bias is not just a technical challenge—it’s an ethical imperative.