A former OpenAI safety researcher makes sense of ChatGPT’s sycophancy and Grok’s South Africa obsession
It has been an odd few weeks for generative AI systems, with ChatGPT suddenly turning sycophantic, and Grok, xAI’s chatbot, becoming obsessed with South Africa. Fast Company spoke to Steven Adler, a former research scientist for OpenAI who until November 2024 led safety-related research and programs for first-time product launches and more-speculative long-term AI systems about both—and what he thinks might have gone wrong. The interview has been edited for length and clarity. What do you make of these two incidents in recent weeks—ChatGPT’s sudden sycophancy and Grok’s South Africa obsession—of AI models going haywire? The high-level thing I make of it is that AI companies are still really struggling with getting AI systems to behave how they want, and that there is a wide gap between the ways that people try to go about this today—whether it’s to give a really precise instruction in the system prompt or feed a model training data or fine-tuning data that you think surely demonstrate the behavior you want there—and reliably getting models to do the things you want and to not do the things you want to avoid. Can they ever get to that point of certainty? I’m not sure. There are some methods that I feel optimistic about—if companies took their time and were not under pressure to really speed through testing. One idea is this paradigm called control, as opposed to alignment. So the idea being, even if your AI “wants” different things than you want, or has different goals than you want, maybe you can recognize that somehow and just stop it from taking certain actions or saying or doing certain things. But that paradigm is not widely adopted at the moment, and so at the moment, I’m pretty pessimistic. What’s stopping it being adopted? Companies are competing on a bunch of dimensions, including user experience, and people want responses faster. There’s the gratifying thing of seeing the AI start to compose its response right away. There’s some real user cost of safety mitigations that go against that. Another aspect is, I’ve written a piece about why it’s so important for AI companies to be really careful about the ways that their leading AI systems are used within the company. If you have engineers using the latest GPT model to write code to improve the company’s security, if a model turns out to be misaligned and wants to break out of the company or do some other thing that undermines security, it now has pretty direct access. So part of the issue today is AI companies, even though they’re using AI in all these sensitive ways, haven’t invested in actually monitoring and understanding how their own employees are using these AI systems, because it adds more friction to their researchers being able to use them for other productive uses. I guess we’ve seen a lower-stakes version of that with Anthropic [where a data scientist working for the company used AI to support their evidence in a court case, which included a hallucinatory reference to an academic article]. I obviously don’t know the specifics. It’s surprising to me that an AI expert would submit testimony or evidence that included hallucinated court cases without having checked it. It isn’t surprising to me that an AI system would hallucinate things like that. These problems are definitely far from solved, which I think points to a reason that it’s important to check them very carefully. You wrote a multi-thousand-word piece on ChatGPT’s sycophancy and what happened. What did happen? I would separate what went wrong initially versus what I found in terms of what still is going wrong. Initially, it seems that OpenAI started using new signals for what direction to push its AI into—or broadly, when users had given the chatbot a thumbs-up, they used this data to make the chatbot behave more in that direction, and it was penalized for thumb-down. And it happens to be that some people really like flattery. In small doses, that’s fine enough. But in aggregate this produced an initial chatbot that was really inclined to blow smoke. The issue with how it became deployed is that OpenAI’s governance around what passes, what evaluations it runs, is not good enough. And in this case, even though they had a goal for their models to not be sycophantic—this is written in the company’s foremost documentation about how their models should behave—they did not actually have any tests for this. What I then found is that even this version that is fixed still behaves in all sorts of weird, unexpected ways. Sometimes it still has these behavioral issues. This is what’s been called sycophancy. Other times it’s now extremely contrarian. It’s gone the other way. What I make of this is it’s really hard to predict what an AI system is going to do. And so for me, the lesson is how important it is to do careful, thorough empirical testing. And what about the Grok incident? The type of thing I would want to understand to assess that is
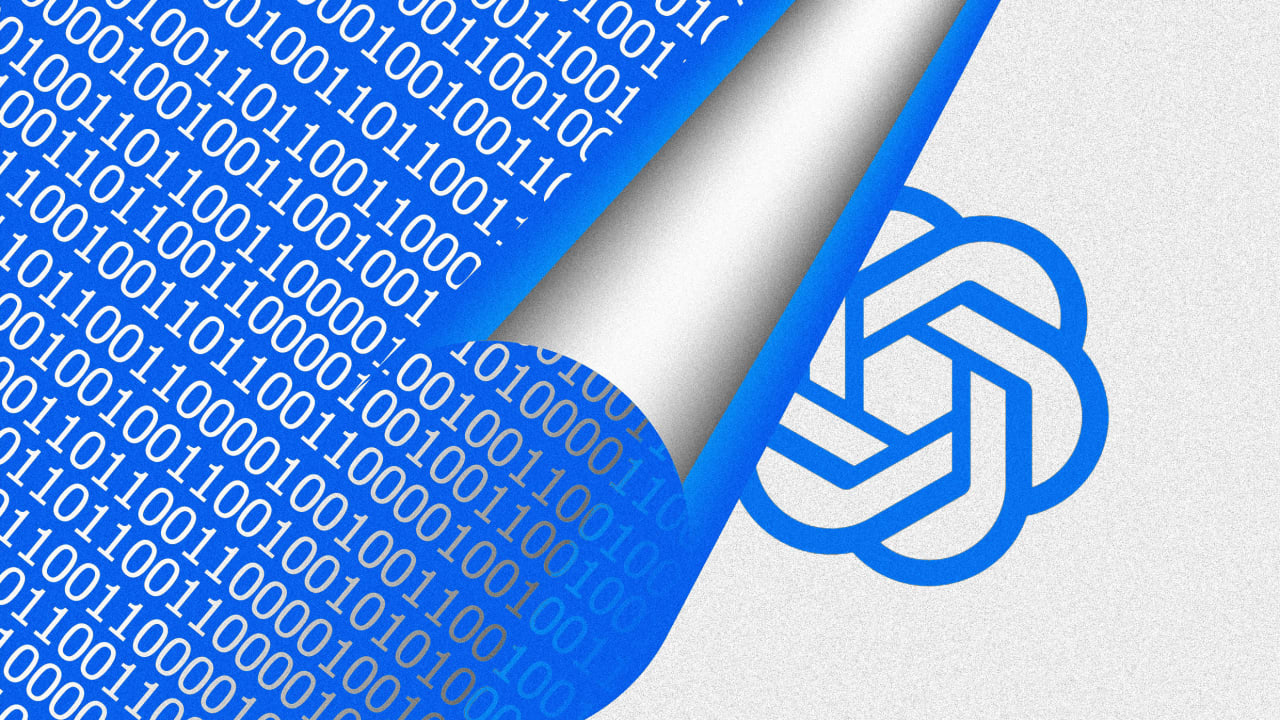
It has been an odd few weeks for generative AI systems, with ChatGPT suddenly turning sycophantic, and Grok, xAI’s chatbot, becoming obsessed with South Africa.
Fast Company spoke to Steven Adler, a former research scientist for OpenAI who until November 2024 led safety-related research and programs for first-time product launches and more-speculative long-term AI systems about both—and what he thinks might have gone wrong.
The interview has been edited for length and clarity.
What do you make of these two incidents in recent weeks—ChatGPT’s sudden sycophancy and Grok’s South Africa obsession—of AI models going haywire?
The high-level thing I make of it is that AI companies are still really struggling with getting AI systems to behave how they want, and that there is a wide gap between the ways that people try to go about this today—whether it’s to give a really precise instruction in the system prompt or feed a model training data or fine-tuning data that you think surely demonstrate the behavior you want there—and reliably getting models to do the things you want and to not do the things you want to avoid.
Can they ever get to that point of certainty?
I’m not sure. There are some methods that I feel optimistic about—if companies took their time and were not under pressure to really speed through testing. One idea is this paradigm called control, as opposed to alignment. So the idea being, even if your AI “wants” different things than you want, or has different goals than you want, maybe you can recognize that somehow and just stop it from taking certain actions or saying or doing certain things. But that paradigm is not widely adopted at the moment, and so at the moment, I’m pretty pessimistic.
What’s stopping it being adopted?
Companies are competing on a bunch of dimensions, including user experience, and people want responses faster. There’s the gratifying thing of seeing the AI start to compose its response right away. There’s some real user cost of safety mitigations that go against that.
Another aspect is, I’ve written a piece about why it’s so important for AI companies to be really careful about the ways that their leading AI systems are used within the company. If you have engineers using the latest GPT model to write code to improve the company’s security, if a model turns out to be misaligned and wants to break out of the company or do some other thing that undermines security, it now has pretty direct access. So part of the issue today is AI companies, even though they’re using AI in all these sensitive ways, haven’t invested in actually monitoring and understanding how their own employees are using these AI systems, because it adds more friction to their researchers being able to use them for other productive uses.
I guess we’ve seen a lower-stakes version of that with Anthropic [where a data scientist working for the company used AI to support their evidence in a court case, which included a hallucinatory reference to an academic article].
I obviously don’t know the specifics. It’s surprising to me that an AI expert would submit testimony or evidence that included hallucinated court cases without having checked it. It isn’t surprising to me that an AI system would hallucinate things like that. These problems are definitely far from solved, which I think points to a reason that it’s important to check them very carefully.
You wrote a multi-thousand-word piece on ChatGPT’s sycophancy and what happened. What did happen?
I would separate what went wrong initially versus what I found in terms of what still is going wrong. Initially, it seems that OpenAI started using new signals for what direction to push its AI into—or broadly, when users had given the chatbot a thumbs-up, they used this data to make the chatbot behave more in that direction, and it was penalized for thumb-down. And it happens to be that some people really like flattery. In small doses, that’s fine enough. But in aggregate this produced an initial chatbot that was really inclined to blow smoke.
The issue with how it became deployed is that OpenAI’s governance around what passes, what evaluations it runs, is not good enough. And in this case, even though they had a goal for their models to not be sycophantic—this is written in the company’s foremost documentation about how their models should behave—they did not actually have any tests for this.
What I then found is that even this version that is fixed still behaves in all sorts of weird, unexpected ways. Sometimes it still has these behavioral issues. This is what’s been called sycophancy. Other times it’s now extremely contrarian. It’s gone the other way. What I make of this is it’s really hard to predict what an AI system is going to do. And so for me, the lesson is how important it is to do careful, thorough empirical testing.
And what about the Grok incident?
The type of thing I would want to understand to assess that is what sources of user feedback Grok collects, and how, if at all, those are used as part of the training process. And in particular, in the case of the South African white-genocide-type statements, are these being put forth by users and the model is agreeing with them? Or to what extent is the model blurting them out on its own, without having been touched?
It seems these small changes can escalate and amplify.
I think the problems today are real and important. I do think they are going to get even harder as AI starts to get used in more and more important domains. So, you know, it’s troubling. If you read the accounts of people having their delusions reinforced by this version of ChatGPT, those are real people. This can be actually quite harmful for them. And ChatGPT is widely used by a lot of people.