10 Urgent AI Takeaways for Leaders
“Despite two years of broad managerial attention and extensive experimentation, we are not seeing the large-scale GenAI-powered business transformations that many people initially envisioned.” Does this statement by MIT SMR authors Melissa Webster and George Westerman reflect your experience? No wonder. After a wild two-year ride of hype, disruption, and experiments for many leaders, you […]
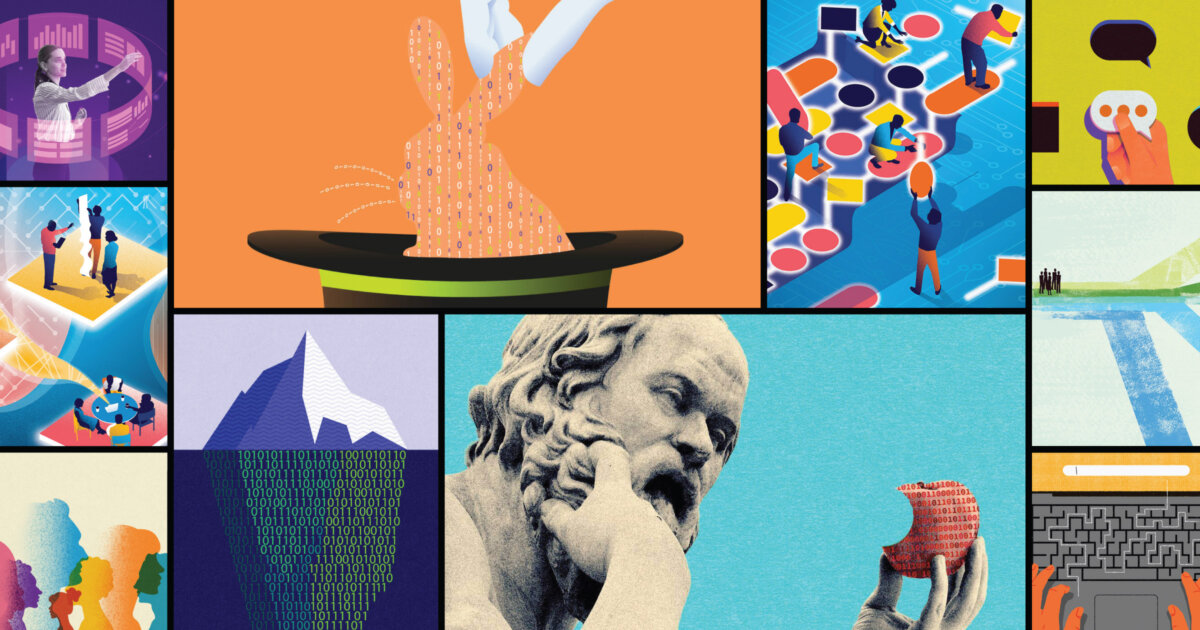
“Despite two years of broad managerial attention and extensive experimentation, we are not seeing the large-scale GenAI-powered business transformations that many people initially envisioned.”
Does this statement by MIT SMR authors Melissa Webster and George Westerman reflect your experience? No wonder. After a wild two-year ride of hype, disruption, and experiments for many leaders, you (and your colleagues) may still be waiting for the big business payoff. You may not have redesigned that critical process, cut time to market, or radically improved customer satisfaction quite yet.
As Westerman, a senior lecturer at the MIT Sloan School of Management, noted in one of our most popular articles on artificial intelligence this year, you may need to practice more patience. The most successful companies are now in the midst of smaller transformations using AI tools — paving the way for that big transformation.
It’s difficult to articulate how hard it is for leaders to shape AI strategy in 2025. After all, this work involves tackling everything from risk management to AI ethics, with some daunting data management and culture challenges thrown in. At the same time, AI and generative AI tools keep evolving. What GenAI tool Claude can’t do this spring, it may well do by summer.
At MIT SMR, we strive to publish fresh, evidence-based advice that leaders can apply at their own organizations. Leaders keenly want guidance on AI strategy: We see this in our article readership data. Here, we’ve gathered 10 of our most popular, valuable AI articles of recent months to share timely lessons on 10 pressing AI issues.
1. Reap GenAI value: Start with “small t” transformations.
“Less than two years ago, generative AI made headlines with its amazing new capabilities: It could engage in conversations; interpret massive amounts of text, audio, or imagery; and even create new documents and artwork. After the fastest technology adoption in history — with over 100 million users in the first two months — businesses in every industry began experimenting with it. Yet, despite two years of broad managerial attention and extensive experimentation, we are not seeing the large-scale GenAI-powered business transformations that many people initially envisioned.
“What happened? Has the technology failed to live up to its promise? Were experts wrong in calling for giant transformations? Have companies been too cautious? The answer to each of those questions is both yes and no. Generative AI is already being used in transformative ways in many companies, just not yet as the driver of a wholesale redesign of major business functions. Business leaders are finding ways to derive real value from large language models (LLMs) without complete replacements of existing business processes. They’re pursuing ‘small t’ transformation, even as they build the foundation for larger transformations to come.”
Read the full article, “Generate Value From GenAI With ‘Small t’ Transformations,” by Melissa Webster and George Westerman.
2. Make smart AI tech-debt trade-offs.
“To understand how today’s business leaders are reinventing their organizations, including the role tech debt plays, Accenture studied 1,500 global companies in 10 countries covering 19 industries and conducted scores of in-depth discussions with C-level leaders. The research found that companies that are well positioned for change have a reinvention-ready ‘digital core’ — a set of key components such as cloud infrastructure, data, and AI that can be easily updated. They also typically set aside around 15% of their IT budgets for tech debt remediation.
“What the research made clear is that today, addressing tech debt is not about eliminating it but managing it. The key lies in knowing what the debt is, what to fix, what to keep, and how to recognize the tech debt that is boosting your company’s innovation capacity.”
Read the full article, “How to Manage Tech Debt in the AI Era,” by Koenraad Schelfaut and Prashant P. Shukla.
3. Unstructured data matters again.
“The great majority of the data that GenAI works with is relatively unstructured, in forms such as text, images, video, and the like. A leader at one large insurance organization recently shared … that 97% of the company’s data was unstructured. Many companies are interested in using GenAI to help manage and provide access to their own data and documents, typically using an approach called retrieval-augmented generation, or RAG. But some companies haven’t worked on their unstructured data much since the days of knowledge management 20 or more years ago. They’ve been focused on structured data — typically rows and columns of numbers from transactional systems.”
Read the full article, “Five Trends in AI and Data Science for 2025,” by Thomas H. Davenport and Randy Bean.
4. AI success requires building a data-driven culture.
“Thanks to the deafening buzz around data science and AI, enterprise leaders no longer doubt the transformative potential of this powerful duo. Yet, a striking statistic reveals a bigger leadership challenge: More than 57% of companies struggle to build a data-driven culture, according to Wavestone research. This indicates that in many cases, leaders believe in the power of data and are investing in AI, but their organizations still aren’t getting the real benefits.
“Indeed, for many leaders, the challenge is not buying advanced analytics tools or building accurate technical solutions. The real hurdle is subtle yet much more important: fostering an environment within an organization where individuals instinctively turn to data anytime they must make a decision. This is the real meaning of being data driven or creating a data culture.”
Read the full article, “Building a Data-Driven Culture: Four Key Elements,” by Ganes Kesari.
5. Philosophy could eat your AI strategy.
“Even as software eats the world and AI gobbles up software, what disrupter appears ready to make a meal of AI? The answer is hiding in plain sight. It challenges business and technology leaders alike to rethink their investment in and relationship with artificial intelligence. There is no escaping this disrupter; it infiltrates the training sets and neural nets of every large language model (LLM) worldwide.
“Philosophy is eating AI: As a discipline, data set, and sensibility, philosophy increasingly determines how digital technologies reason, predict, create, generate, and innovate. The critical enterprise challenge is whether leaders will possess the self-awareness and rigor to use philosophy as a resource for creating value with AI or default to tacit, unarticulated philosophical principles for their AI deployments. Either way — for better and worse — philosophy eats AI. For strategy-conscious executives, that metaphor needs to be top of mind.”
Read the full article, “Philosophy Eats AI,” by Michael Schrage and David Kiron.
6. GenAI can turbocharge how organizations learn.
“Combined with traditional AI, generative AI expands the scope of potential improvement in many processes and decisions and the ease with which this new knowledge can be applied. This, in turn, creates the potential for a positive compounding effect on organizational learning, with human and machine agents working in concert to create new competitive advantages.”
Read the full article, “Turbocharging Organizational Learning With GenAI,” by Paul Baier and John J. Sviokla.
7. GenAI versus analytical AI: Pick your projects wisely.
“Leaders should recognize that generative AI and analytical AI [tools] are complementary rather than interchangeable. GenAI focuses on efficiency and automation, like using AI-powered chatbots to increase call center productivity, whereas analytical AI enhances strategic decision-making, like determining the best time or offer for each customer contacted by the call center.
“Which flavor of AI suits your business problem best? A critical step in creating a road map for AI projects involves conducting ideation workshops in which business and technical leaders can brainstorm about opportunities to apply AI to organizational challenges.”
Read the full article, “Analytical AI: A Better Way to Identify the Right AI Projects,” by Pedro Amorim, Teresa Bianchi de Aguiar, Luís Guimarães, Bernardo Almada-Lobo, and Bruno Teixeira.
8. Bring your own AI (BYOAI) can’t be banned.
“Given the risks associated with BYOAI, it may seem logical for leaders to consider banning unvetted GenAI tools outright. The prospect of uncontrolled GenAI use, combined with the uncertainty around legal and regulatory exposure, can understandably make leaders cautious. However, the executives we interviewed said that banning BYOAI is neither practical nor effective. Employees — especially those already feeling stretched thin — often turn to GenAI tools to enhance their personal productivity. Restricting access only pushes them to find unofficial workarounds, potentially bypassing established governance frameworks. This can ultimately amplify the very risks leaders aim to mitigate.
“Moreover, a ban will prevent employees from exploring new use cases, blocking opportunities for creative problem-solving and the discovery of potential efficiency gains across the organization.”
Read the full article, “Bring Your Own AI: How to Balance Risks and Innovation,” by Nick van der Meulen and Barbara H. Wixom.
9. Pay more attention to GenAI app evaluation.
“An evaluation process consists of evals — automated tests designed to measure how well your LLM application performs on metrics that capture what end users care about and what is important to the business. Evals speed development by focusing effort on the areas that matter and increase the likelihood of building applications that deliver organizational value. However, the reality is that many teams underinvest in evals. The result: uneven progress and, ultimately, canceled GenAI projects or flawed applications that fail to achieve the business goal.
“Business leaders, IT leaders, and developers working hand in hand to develop generative AI apps to solve business problems all benefit from a strong evaluation process. The business and IT leaders gain visibility into the app’s true quality level over the course of the development cycle, and developers can answer critical questions, like ‘Are we making enough progress?’ or ‘What should the next dev cycle focus on?’ or ‘Is the application “good enough” to deploy?’”
Read the full article, “The GenAI App Step You’re Skimping On: Evaluations,” by Rama Ramakrishnan.
10. What-if questions call for a new machine learning tool.
“Causal ML — an emerging area of machine learning — can help to answer … what-if questions through causal inference. Similar to how marketers use A/B tests to infer which of two ads is likely to generate more sales, causal ML can inform what might happen if managers were to take a particular action.
“This makes the technology useful in many of the same business functions that use traditional ML, including product development, manufacturing, finance, human resources, and marketing. Traditional ML is still the go-to approach when the only goal is to make predictions — such as whether stock prices will rise or which products customers are most likely to buy. When a company wants to predict what would happen if it were to make one decision versus another — such as whether a 10% discount or none is more likely to induce a customer to make a repeat purchase — it needs causal ML.”
Read the full article, “A New Machine Learning Approach Answers What-If Questions,” by Stefan Feuerriegel, Yash Raj Shrestha, and Georg von Krogh.