Speed, Ease, and Expertise With AI: Lenovo’s Linda Yao
Linda Yao, chief operating officer and head of strategy for Lenovo’s Strategy, Solutions, and Services Group and vice president of hybrid cloud and AI solutions, joins the Me, Myself, and AI podcast to explain the organization’s transition from technology product company to managed services provider. It’s now helping organizations with the change management required to […]
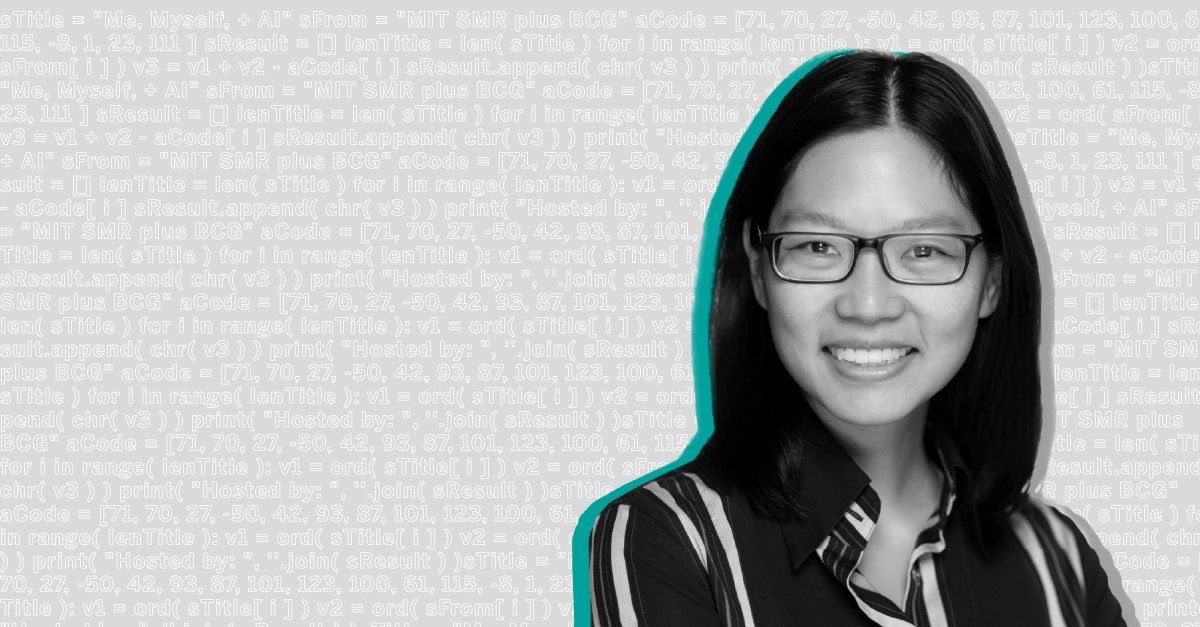
Linda Yao, chief operating officer and head of strategy for Lenovo’s Strategy, Solutions, and Services Group and vice president of hybrid cloud and AI solutions, joins the Me, Myself, and AI podcast to explain the organization’s transition from technology product company to managed services provider. It’s now helping organizations with the change management required to implement AI in the enterprise.
She shares both a framework around speed, ease, and expertise to facilitate this adoption, as well as the four pillars of AI readiness that Lenovo guides its clients to achieve. Tune in to this episode, also, for Linda’s perspective on the role of human connection in what she calls the era of inference, a time when we should focus on the implementation of maturing AI tools.
Subscribe to Me, Myself, and AI on Apple Podcasts or Spotify.
Transcript
Shervin Khodabandeh: Stay tuned after today’s episode to hear Sam and I break down the key points made by our guest.
What are the four areas that companies need to prioritize for AI readiness? Find out on today’s episode.
Linda Yao: I’m Linda Yao from Lenovo, and you’re listening to Me, Myself, and AI.
Sam Ransbotham: Welcome to Me, Myself, and AI, a podcast on artificial intelligence in business. Each episode, we introduce you to someone innovating with AI. I’m Sam Ransbotham, professor of analytics at Boston College. I’m also the AI and business strategy guest editor at MIT Sloan Management Review.
Shervin Khodabandeh: And I’m Shervin Khodabandeh, senior partner with BCG and one of the leaders of our AI business. Together, MIT SMR and BCG have been researching and publishing on AI since 2017, interviewing hundreds of practitioners and surveying thousands of companies on what it takes to build and to deploy and scale AI capabilities, and really transform the way organizations operate.
Sam Ransbotham: Hey, everyone. Thanks for joining us. Today, Shervin and I are talking with Linda Yao from Lenovo. At Lenovo, Linda is the vice president of AI solutions and services as well as the chief operating officer and head of strategy for [Lenovo’s] Solutions and Services Group. Linda, thanks for joining us.
Linda Yao: Thanks so much, Sam. It’s great to be here.
Sam Ransbotham: All right, let’s start with Lenovo. For our listeners who are not familiar with Lenovo, can you give us a brief overview?
Linda Yao: Yes. Lenovo is a global technology company. We operate in 180 markets. We’re part of the Global Fortune 500. We started, many decades ago, as a PC manufacturer, and then … we acquired, in 2005, the IBM PC division and then decided to diversify our technology business.
We acquired the x86 data center infrastructure business from IBM. We acquired the Motorola phones business from Google after they had bought Motorola. And with that, we created what we call our end-to-end, pocket-to-cloud portfolio.
The latest journey that we’ve been on the past three to five years has been all about service-led transformation. Lenovo just launched — three and a half years ago — our Solutions and Services Group, which is all around moving up the stack and answering the demand from our customers to help them, not just with their hardware technology needs but also with their software, their services and solutions, and generating those customer outcomes.
Sam Ransbotham: So I’m guessing artificial intelligence ends up being a big part of those solutions and services. Tell us about that.
Linda Yao: Absolutely. I’m probably dating myself here, but this has been the third wave of AI to hit the enterprise since I’ve been working in technology. The first wave about 20 years ago was when big data really began finding itself in enterprise commercialized applications, most notably high-frequency trading.
Then we fast-forward 10 years to about a decade ago, and that was around the time when AWS [Amazon Web Services] really made computing more accessible and democratized. And so we had a way to use that big data. At that time, every enterprise was looking at setting up its own machine learning team. Every university was trying to launch a data science master’s program.
That was where I really saw these types of data science, machine learning, and AI applications hit the workforce and use this technology to make our workflows more efficient and generate more and more of those business results. But it was still quite niche, so what really excites me about the past couple of years is the fact that generative AI [GenAI] has captured the imagination. You know, the majority of AI applications that we work on today are not necessarily generative, but what ChatGPT did a couple of years ago was really put AI at the fingertips of every consumer and really put it into the mental model of every C-suite member, so it’s become a little bit of the rising tide that raises all of our boats when it comes to technology adoption. [It’s] super exciting. And that is why at Lenovo, I’m very excited and proud to be launching the AI solutions practice.
Shervin Khodabandeh: Tell us more about that. What does that practice do?
Linda Yao: We really have embraced AI from an enterprise, commercial, and consumer perspective in a couple of different ways. The first is by embedding AI into all of our existing offerings. I will give you an example: We provide digital workplace solutions. We help our customers and their enterprises and organizations provide support around end user devices. What we’ve been able to do now with generative AI is to hyper-personalize. If you would have asked me five years ago to tailor a company’s IT support desk to help specific employee profiles, even down to the individual user patterns on their device, could I have done it? Probably, but could I have done it in a cost-effective way? Probably not. Because I would have had to hire a bunch of analysts and some delivery hub somewhere to really analyze and go through the data of every user’s patterns.
What we’ve launched now with this AI solutions practice is a set of services — advisory, professional, and managed services — to help companies also adopt AI. That includes the change management services to help companies really assess whether their people are ready, whether they have the training in place, all the way to technology integration and implementation services to ensure that you have the right GPU access and configurations.
Sam Ransbotham: You’ve got a recent study that … is talking about how challenging CIOs, in particular, are finding handling this current wave of artificial intelligence intention, I guess. You pointed out that it’s brought a lot of attention, but with that comes some difficulties for the C-suite. I think one of the statistics that caught my eye, you said something about 96% of CIOs anticipate increasing budget, which doesn’t surprise me, but 40-something percent found that people were struggling to see ROI from AI investments or didn’t expect it for two or three years.
Why are people putting that money in if they’re not getting ROI?
Linda Yao: It’s a great question, Sam, and you’re referring to our survey that we did for CIOs and IT decision makers across many markets, not limited to any particular geographic location. But what we found is similar statistics across each of these markets.
Every CIO has some impetus to want to embrace this technology and really put it to work in the enterprise, but you’re absolutely correct. Fewer than half of them see quick ROI or see ROI on the horizon or see ROI that’s in a very achievable, certain way.
We identify three necessary items that CIOs are looking for. We call it speed, ease, and expertise. Speed means that we have a set of offerings that we call the AI Fast Start. Within one fiscal quarter, within 90 days, whether it is an enterprise AI problem or an end user AI problem, we can help a customer to spin up a proof of concept and a proof of value so that they can use this to demonstrate to their stakeholders why [they should] continue the experiment or why to launch into production or why to deploy at scale.
From an ease component, we have a good sense of what works and what works less well in an enterprise setting. And we have curated a lot of those best practices by domain, by function, into what we call an AI Library.
And then, finally, is the expertise. A lot of AI, when you get beneath the use case to actually make it run and to make it run at scale, it is all about GPU configurations. It is all about data access. It is all about the engineering workflows. So that level of expertise, that level of depth, is also what we’re able to contribute.
Sam Ransbotham: One of the things that I think is interesting here is that with everybody paying so much attention to artificial intelligence, one of the things that your study mentioned was the risk of AI washing. I don’t think we’ve talked about AI washing much yet on this show, and this seems like a great opportunity to mention that.
It seems like, with everybody saying, “Oh yeah, AI is everything,” there’s a tendency to put a sticker on every solution and call it “new and improved, now with even more AI,” when, maybe if you peel back the layers, there’s not quite as much artificial intelligence under there as you might expect. What’s the root of all this AI washing? Is it a problem?
Linda Yao: You know, Sam, AI washing is a little bit inspired by the word greenwashing where a lot of companies might, you know, with good intentions, attribute a lot of activities to being more environmentally sustainable or heading toward their ESG [environmental, social, and governance] goals and maybe get a little bit carried away or maybe a little bit exaggerated. We find the same thing on AI washing, in the sense that everyone is so excited to embrace AI. And there’s quite a level of FOMO [fear of missing out] as well.
You know, these CIOs, these C-suite members, they don’t want to miss out on the trend. We want to show that we’re making progress. We want to show that as AI and GenAI have hit the public mindset that we as enterprises are also using it to improve our businesses. And so, sometimes what happens is that a lot of things and a lot of solutions might get branded as AI, but, to your point, it might start from a kernel of truth but might not be the whole truth. It’s not necessarily done nefariously. I think it’s just because everyone’s so excited, but not everyone has come up to speed yet on the latest technology, what it can actually do and what AI can’t do, and how quickly, or what it takes to implement it.
We have found that the best defense against AI washing is really just to look at the facts, to look at results, because results don’t lie. And when we look at the results that we’ve actually achieved with AI, we are able to really dissect that solution down to: What are the algorithms? Where did we access the data? What is the computational metric that we used to measure this ROI? And what types of applications and what types of business scenarios do we use it in?
I’ll give you a couple of examples of our own early adopters of GenAI. When I look at our own C-suite, our chief marketing officer and our chief legal officer were two of the first big functions who saw GenAI as a way to capture a lot of the lower-hanging fruit in terms of their automation needs. What we were able to do for our chief marketing officer was to create an AI-powered content generation platform that is now able to create tailored content for our … product brochures, customer pitch books, feature fact sets — create all of that in a way that has now saved them 90% on third-party agency costs. That is the type of result that doesn’t lie. That is how we know that this is not an AI wash. This is actually hitting the bottom line.
Shervin Khodabandeh: I just want to maybe riff on this a little bit because you mentioned the three unlocks to ROI: speed, ease, and expertise. But it was also very much, I would say, tech-focused because you’re talking to the CIO, I would imagine. The part that I’ve seen a lot of AI programs fail on the ROI side is … they can’t even get off the ground without those three things that you said, right? Because you’re going to build stuff that’s not going to run or not going to run at scale, or the data is going to be all over the place, or they don’t have enough compute power, so all of that is just a completely necessary condition.
But then so many proofs of concepts just sort of sit on the shelf because [of] the organizational aspect of the change that’s required and people doing things differently. You mentioned the marketing example for Lenovo, which was quite successful from what you’re describing. But there you’re inside the company and so you’re getting to affect how marketers and brand and creative people are doing things. How do you traverse the gap between the tech side and the actual usage or as, you know, Sam and I talk about, from the production to the consumption?
Linda Yao: I really think that a large part of it is the people element, to your point, in addition to the technology element. Our methodology is really centered on four pillars of readiness. So those four pillars of readiness are security and data, people, technology, and processes. It sounds pretty simple on paper, but what we’ve noticed is exactly your point, Shervin, that oftentimes, when we look at the Maslow’s hierarchy of needs, the first hurdle that these stakeholders have to overcome is all around security and data. They want to understand, at the very basic level, “How is AI and GenAI going to impact my security posture? Is my data ready? Do I have the right guardrails up? Do I have the right firewalls up?”
Once we get past that point of what I would call risk management, the next thing they want to know is if their people are ready. That’s why, as part of our AI practice, change management and AI adoption and training [are] a huge aspect of it. A lot of what we do in this people readiness assessment is also help a company understand which of [its] departments are most ready to embrace AI. Which job profiles, which job tasks in that department might be more likely to embrace AI, see the gains, and then be a positive feedback loop and a beacon for the rest of the organization?
We find that one of the biggest barriers to scaling AI, outside of technology, is actually the people and understanding, as an example, if the AI is meant to augment their tasks or to replace some of their tasks. And then we have technology and processes, right? Technology, processes, and data [are] still by far the biggest barrier for CIOs. [They are] the majority of what they see when it comes to inhibitors to scaling AI.
Sam Ransbotham: When I hear these, they all make sense to me and they resonate. The idea of security and people and tech and processes make sense, but there’s something about them that does not feel very AI specific. That feels like the same sorts of things we’ve had with IT implementations for years and years. Part of that’s comforting because we, in theory, should have experience with this, but is there anything different about artificial intelligence with this process that differs from the prior generations?
Linda Yao: It’s incredibly astute, Sam, because when we develop these four pillars of AI readiness, it’s actually the same areas and the same categories we looked at for our digital transformation that has been occurring for decades and [is] not limited to AI and certainly not limited to GenAI. I think what GenAI though has really brought to the forefront is more focus on each of these pillars in a different way.
For example, the security question: It is so much more prevalent now just because of the sheer amount of data that AI requires and the amount of data that AI now generates. Understanding where the data lies, where it’s being accessed, how we govern it, what types of barriers we put around the usage of that data and around the newly generated data that becomes hyper-important.
Shervin Khodabandeh: Linda, you’ve been doing this for some time, so you have some longitudinal perspective here as well. Do you see in the data since the advent of generative AI that CIOs and companies, more broadly, have a better sense of where they’re going with AI or a worse sense of where they were going with AI?
Because Sam and I did some research here. The reason I’m asking is there was a time where [we] would ask thousands of folks that we surveyed, “Do you have an AI strategy?” And back in 2017 and 2018, 20%, 30%, 40% would say, “Yes, but we’re working on it.” By 2018, 2019, 2020, most people would say, “Yes, we have a strategy.” And by 2021, 2022, most companies would have an AI strategy, and they were investing in [it] and they were getting some results. But [in] 2023, 2024, what we saw in the data was a smaller percentage would say, “Yes, we really, really know what we’re doing with AI.”
And I’m wondering, how does it affect the CIO’s outlook from where you’re sitting in the future, in terms of how well articulated the vision and the plan and the direction [are]?
Linda Yao: That’s such an interesting question. AI strategy is a topic where the level of precision and certainty that we might see in some other ways of technology adoption is not necessarily there. But I don’t see that as a bad thing. Before, I mentioned the couple of waves of AI adoption in the enterprise that I’ve experienced even in my career. Before, they were more targeted, right? Before, it was not every department that had the ability to generate and curate so much data to be the fuel for AI.
Before, not every organization had the skill set necessary to be developing data science algorithms, to be implementing machine learning engineering applications, and to be taking those things to scale. It really is at our fingertips. The barriers to entry for every end user, for every consumer, have really been lowered — in terms of the types of AI we can experiment with, the types of AI and data that we can create ourselves, and then, therefore, how quickly it can proliferate. But I do think that one huge weapon in the arsenal of these C-suite members is that AI is now so accessible, it has captured the imagination. We can each now become more and more educated on AI ourselves.
Shervin Khodabandeh: Linda, we have a segment here that we call five questions. I’m going to ask you five questions, and you tell me the first thing that comes to your mind.
Linda Yao: Word association. Bring it on.
Shervin Khodabandeh: What do you see as the biggest opportunity with AI right now?
Linda Yao: I think that one of the biggest opportunities I see with AI right now is how we are now moving from the era of training to the era of inference, right? What I mean by that is we have spent a lot of time and a lot of investment now honing the tools, honing the foundation models, honing the platforms to really harness all this power of artificial intelligence. Now, I think we’re going to see a real renaissance and resurgence around how do we actually put that to use? What are the applications going to be? What are the use cases going to be? And how is that going to make a real difference for you and me?
Sam Ransbotham: That’s nice.
Shervin Khodabandeh: What is the biggest misconception with AI?
Linda Yao: I think one of the biggest misconceptions is, again, this trepidation about AI coming for our jobs, AI coming for our lives, AI coming for our consciousnesses. Maybe I’m too optimistic, and I like to believe in a world where we cannot just coexist but actually wield AI to make ourselves better and to make society and industry better. To me, that’s a big misconception, that somehow we as humans will lack the ability and somehow be overcome by this AI that we create. No, I think we just have to go about it responsibly and intelligently.
Shervin Khodabandeh: What was the first career you wanted? What did you want to be when you grew up?
Linda Yao: I wanted to be an airplane pilot. I wanted to fly fighter jets, and that’s actually one of the reasons that led me to my previous employer.
Sam Ransbotham: You were at Boeing before.
Linda Yao: I was at Boeing before, and I was able to work on the flight line for the F-18s, and it was one of the highlights of my career there.
Shervin Khodabandeh: Wonderful. When is there too much AI?
Linda Yao: When is there too much AI? You know, there are a lot of applications now that really enforce the human in the loop. And I think the human in the loop is a way to bring in another guardrail that really focuses on keeping AI within the bounds of where it’s meant to be. I don’t want to make that sound too sinister, right? Like, the AI is a naughty creature that’s always trying to get out, but there are definitely use cases where AI is not the applicable technology, right? In use cases where we don’t have the right data or enough data or an unbiased view of the data, those are the use cases where AI is not going to work well because data is the fuel for AI. So we’ve known for ages now, in any type of technology, garbage in, garbage out. And that’s where we have to really be careful.
Shervin Khodabandeh: What is the one thing you wish AI could do right now that it can’t?
Linda Yao: You know, for AI — I think it’s actually coming soon — I really wish that AI could become even more hyper-personalized [and] really help each of us as individuals, not just make ourselves more productive at work but really make ourselves more, you know, fulsome and more engaged in our lives outside of work, with our families. Closing those connection loops, really closing the distance and some of those barriers.
I think that element of AI that helps us become better — I will say, emotional-sensing perceptive people — is probably coming, to be honest. I see the level of hyper-personalization. I see the advancements we’re making in AI feeling and sensing and perceiving more around us. I think it will come, but getting past the AI that’s all around cold, hard facts and results, and getting to something that is a little more holistic, that’s something I wish AI could do now.
Sam Ransbotham: Linda, thanks for joining us. That feels like a great way to end — thinking about how hyper-personalization can help us make connections with people and not with technology. Thanks for taking the time to join us.
Linda Yao: Thank you for having me, Sam and Shervin.
Sam Ransbotham: We just finished talking with Linda, and a couple interesting points came out: the emphasis on the ROI and the difficulty, and maybe the short-term horizon for ROI, but also the conversation went in a little different direction. While we started off talking about organizations and technology, we ended up talking about people and human connections. Both of those are kind of interesting.
Shervin Khodabandeh: It’s interesting Linda’s commenting on that, too, because it is a fact that before generative AI, companies were spending quite a lot on AI, and then 2023 and 2024 came, and then they doubled down [on] those investments. They got a pass in 2023 and 2024 in terms of showing returns, particularly some of … this AI washing thing that she was talking about, which is like, “Oh, no, it’s important. We’ve got to do it.” This notion of AI washing is similar to greenwashing, so [it] totally makes sense that investments went in [and some achieved] great returns. We see a mixed bag, right?
I think that’s also a real thing that they’re seeing from their lens. Sam, you pointed out that this seems like a digital transformation kind of a thing, so what’s different? I think that it’s got to be different because the patience isn’t there. The rate of change isn’t the same. And I think a lot of it is in the feedback loops and the learning loops and trying faster and learning faster and changing the nature of work. That was the main thing for me.
Sam Ransbotham: I think if I was sitting in a CIO suite and like you said, “Oh, you get a pass for 2023, 2024.” Well, how many more passes do we get? I feel like in some sense we’re saying, “Oh, no, this time give us a couple of years. This time will pay off.” How many times can we reach out and make that promise?
Shervin Khodabandeh: Exactly. I think this is going to be a tough year: 2025 and 2026 are going to be tough years for companies, and they need to show impact.
The other thing I really liked, which was also how we ended the show, was on this notion of AI as a way for us to be more human, which is a little bit of doing a little bit of jujitsu on AI itself, right?
I think she’s right. It’s not that far off with everything that multimodal can do with tone and language and context and all that. It can be a coach. I mean, technically, it can be a coach and help us understand ourselves better, be more aware, understand the impacts that we have with our words and on other people. Minimally, it could remind us to pick up the phone and call the folks we haven’t called. It’s interesting.
Sam Ransbotham: That feels like AI washing. That doesn’t need to be AI.
Shervin Khodabandeh: What do you think about that, though, Sam?
Sam Ransbotham: I mean, part of me wonders sometimes if we are searching so hard for there to be a human connection that we grasp at straws. But on the other hand, you’re right, that does feel like a potential use, that there’s no reason to believe that AI use can only affect this part of our lives and not other parts of our lives. It would be sort of naive to think that it would be limited in scope to just our workplace efficiency. I have some hope for that.
Shervin Khodabandeh: But I could see it can go in scary ways.
Sam Ransbotham: I do worry that it starts to feel [inauthentic] and [disingenuous] that, you know, if I’m being nice to you, Shervin, but only because I’ve got a … red light showing in my monitor that my tone needs to improve when I’m talking to you, you’re going to see right through that. You’re going to know that I’m only doing it to pass my content filter. That doesn’t feel authentic. That seems like the technology is putting [up] a barrier.
Shervin Khodabandeh: Exactly.
Thanks for listening, everyone. Next time, Sam and I speak with Chandra Kapireddy from Truist. Please join us.
Allison Ryder: Thanks for listening to Me, Myself, and AI. Our show is able to continue, in large part, due to listener support. Your streams and downloads make a big difference. If you have a moment, please consider leaving us an Apple Podcasts review or a rating on Spotify. And share our show with others you think might find it interesting and helpful.